In today’s fast-paced business landscape, data analytics has emerged as a cornerstone for informed decision-making and driving growth. However, several challenges can impede the scaling of data analytics initiatives within an enterprise. From grappling with legacy systems that lack compatibility to establishing robust data governance frameworks, and from facing cultural resistance to ensuring data quality and measuring the return on investment (ROI) of data analytics projects, organizations often encounter roadblocks on their path to success.
In this article, I identify top obstacles and offer practical and effective solutions to help enterprises overcome them. By taking a proactive approach to confront these challenges, you can unlock the true potential of your data and analytics programs, enabling smarter, data-driven decision-making, and propelling your business towards unprecedented growth and success.
Here are the five most significant challenges when it comes to scaling data analytics with an Enterprise:
-
Outdated Systems – Scaling data and analytics in numerous enterprises is hindered by obsolete legacy systems. These systems present inflexibility, high maintenance costs, and an inability to support modern analytics tools. Consequently, data engineers encounter significant challenges when attempting to derive insights from the data.
The remedy lies in modernizing the legacy systems. Enterprises should consider migrating their data to cloud-based systems or embracing agile applications that seamlessly integrate with modern analytics tools. This approach streamlines data extraction, enhances scalability, and empowers data engineers to extract insights from the data with greater efficiency.
-
Ineffective Data Governance – In any enterprise, data governance plays a vital role in scaling data and analytics. It encompasses the establishment of policies, procedures, and standards to ensure data integrity, availability, and security. Proper implementation of data governance is paramount, as it safeguards against storing and utilizing incorrect data, which could result in flawed analyses.
To achieve an effective data governance framework, clear communication of governance policies and procedures to all stakeholders is essential. Additionally, these policies and procedures should be customized to suit the unique needs of the enterprise, while defining the roles and responsibilities of various departments.
-
Cultural Resistance – Enterprises may encounter employee resistance while attempting to scale data and analytics, as some employees view it as a threat to their job security. Additionally, resistance to change can emerge due to a lack of buy-in from senior management.
To foster employee buy-in, it is important to educate them about the advantages of embracing data and analytics solutions within the enterprise. Providing training and education on the latest technology and techniques can alleviate concerns and reinforce the benefits of the initiative. Furthermore, demonstrating leadership through a top-down approach, where senior management leads by example and showcases effective data and analytics utilization, can inspire confidence and acceptance among the employees.
-
ROI Measurement Challenges – Measuring the return on investment (ROI) for expanding data and analytics initiatives poses a considerable hurdle, particularly when the initial investment is perceived as a fixed cost. This perception can make it challenging to obtain funding or allocate resources for future scaling efforts.
To gauge ROI efficiently, businesses must prioritize the assessment of how data and analytics initiatives directly influence their overall business outcomes. Measuring metrics like operational efficiencies, revenue growth, and cost savings can demonstrate the ROI of data and analytics initiatives. Furthermore, by conducting regular assessments, organizations can pinpoint areas requiring improvement and use this insight to guide their future investments in D&A initiatives.
-
Poor Data Quality – Ensuring data quality is essential for the success of data and analytics initiatives. Insufficient data quality can result in misleading analyses, inaccurate insights, and potentially lead to legal or financial consequences for the enterprise. These data quality issues may arise due to inconsistent data, inaccuracies, or other data quality control challenges.
To guarantee data quality, the enterprise must implement data quality control procedures and conduct regular checks to ensure that the data meets rigorous standards. Additionally, investing in data quality management technologies can enhance the efficiency of data quality control procedures, further bolstering the overall data quality.
In Summary…
Scaling data and analytics within the enterprise presents its share of obstacles, but with focused efforts, it is attainable. Enterprises must make strategic investments in modern technologies, create well-defined governance policies, offer comprehensive training and education, accurately measure ROI, and implement effective data quality control procedures to achieve successful scaling. Addressing the needs of all stakeholders and departments is crucial throughout this process. By following these steps and ensuring alignment with various stakeholders, data and analytics can become a powerful tool that facilitates business growth.
Recent Blogs
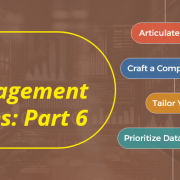
Essential Tips for Telling Data Stories to Executives: Part 6 of Data Management Blog Series
July 24, 2024
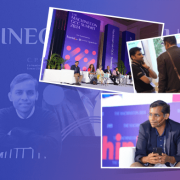
Global Capability Centers Lead the Way in AI Innovation: Highlights from 2024 GCC Summit
July 18, 2024
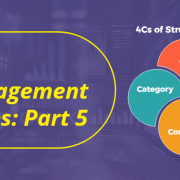
4Cs of Structured Data: Part 5 of Data Management Blog Series
July 11, 2024
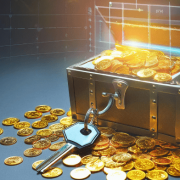
Full Stack Data Products: Bridging the Insight-to-Action Gap
July 9, 2024