A Machine Learning and Operations (MLOps) platform plays a critical role in enabling data scientists to develop and train models to fulfill business priorities. The key asks business leaders have from their data science teams are centered on driving better sales and customer engagement. The MLOps platform provides the environment data scientists need to create and train models that achieve specific business objectives. Platform developers have a wide variety of artificial intelligence (AI) and machine learning (ML) technologies at their disposal. While such a platform lays the foundation for building data science models that can give businesses a disruptive advantage, developers have to comprehend with a problem of plenty.
How to Compose a Harmonious Tool Stack?
Getting out of the abundance syndrome requires developers to tap into their reverse engineering skills. They have to take stock of the key challenges faced by the business to shortlist technologies that can overcome them. Putting the right tool stack together then becomes a matter of matching must-have MLOps platform features with business priorities.
Characteristics of a Robust MLOps Platform
We have listed below few of the many characteristics of a robust MLOps platform.
- Scalability
With a number of data scientists and ML engineers tinkering with multiple models at any given point of time, platform scalability is essential. If the platform is unable to support multiple users, their collective efforts to improve ML algorithms and codes will create a drag and reduce team productivity. - Version Control
The iterative nature of the tasks performed by data scientists requires them to test multiple models, optimize parameters, and tune features while dealing with a vast amount of data. Data science teams can’t be efficient if they cannot track model versions with changes to parameters, code, and data. Version control frameworks and Git repositories provide the means for tracking model versions and their performance. - Data and Concept Drift Sensitivity
With the passage of time, data and concept drifts become inevitable leading to inaccurate results. Models can be trained to trigger training and retraining routines when they detect drift patterns in data. - A/B Testing
The ability to conduct A/B testing is the cornerstone of developing effective data science models. An ideal MLOps platform should enable data science teams to put their models to test with different sets of users. It enables them to deploy models that are either at par with existing models or better than them.
Build an MLOps Platform Your Data Science Team Deserves
In this advisory note – we have defined effective strategies to build a robust and scalable MLOps platform.
Recent Blogs
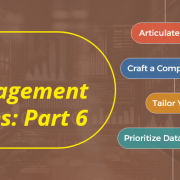
Essential Tips for Telling Data Stories to Executives: Part 6 of Data Management Blog Series
July 24, 2024
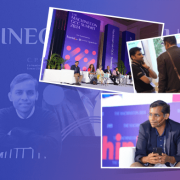
Global Capability Centers Lead the Way in AI Innovation: Highlights from 2024 GCC Summit
July 18, 2024
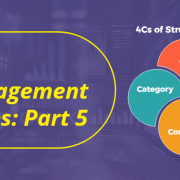
4Cs of Structured Data: Part 5 of Data Management Blog Series
July 11, 2024
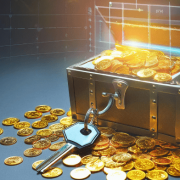
Full Stack Data Products: Bridging the Insight-to-Action Gap
July 9, 2024