Reliability is one of the foundations of trust when it comes to effective artificial intelligence (AI) systems. Without it, user trust can be swiftly eroded, bringing into question any beneficial outcomes. Here, we discuss five key facets of reliability within an AI framework:
Monitoring and Alerts in the World of AI
The heartbeat of an AI system, much like in biological creatures, can indicate when things are functioning well, or when conditions might be headed towards critical states. By embedding monitoring protocols into AI systems, we can alert human supervisors when outputs deviate from expected norms. Consider the analogy of a self-driving car equipped with a system that triggers a warning when the vehicle encounters circumstances that deviate from acceptable parameters, such as a sudden change in weather. In an AI context, machine learning models that form the core of many AI applications can deviate from their training when they encounter data significantly different from the data on which they were trained. In this case, monitoring and alert systems could provide early indicators of ‘drift’ in model performance, allowing human supervisors to intervene swiftly when required.
Contingency Planning
Contingency planning is akin to having a well-rehearsed emergency protocol that guides actions when errors occur in the system. Under the hood of many industry-leading AI systems, contingency plans often take the form of fallback procedures or key decision points that can redirect system functionality or hand control back to human operators when necessary. In healthcare AI, for example, contingency planning might involve supplementary diagnostic methods if the AI system registers an unexpected prognostic output. It is critical to pre-empt potential failings of an AI system, charting a path ahead of the time that enables us to respond effectively when the unexpected occurs.
Trust and Assurance
Trust, that ethereal quality, is not a one-time establishment in AI systems but an ongoing, ever-refreshing assurance to users about the system’s reliability. A banking AI application, for example, would be challenged to win over customers if it didn’t consistently meet or exceed their expectations. To establish trust, AI systems should reliably function within their intended parameters. Regular testing and validation of the AI modules can ensure the system’s dependable service and promote users’ confidence. When users witness first-hand the system’s performance and responsiveness to their needs, trust is reinforced. In this delicate arena, transparency about system operations and limitations contributes significantly towards nurturing user trust, maintaining the relationship with the technology and its human benefactors.
Audit Trails
Audit trails are like breadcrumbs, revealing the steps taken by the AI system in reaching a conclusion. They offer transparency and facilitate interpretation, helping users to understand complex decision-making processes. In a legal AI system, for example, providing justifications for case predictions can foster trust by making the technology more approachable. Moreover, audit trails enable accountability, a fundamental principle for responsible AI. They allow us to trace any systemic malfunctioning or erroneous decision-making back to their origins, offering opportunities to rectify faults and prevent recurrence.
Data Quality
Data quality is the compass by which AI systems navigate. Low-quality data can lead our intelligent systems astray, sabotaging their expected performance and reliability. Ensuring data quality involves careful curation, detangling biases, removing errors, and confirming the data’s relevance to the problem at hand. Take environmental AI, for instance, where data such as climate patterns, pollution levels, and energy consumption form inputs to predictive models forecasting weather changes. If the quality of data is poor in any measurement, the forecasts – and so the reliability – of the AI system are at stake. Therefore, consistent checks and validation processes should be conducted to maintain the credibility of the data, underpinning the reliability of the whole system.
In essence, reliability in AI is a holistic exercise underpinned by vigilant monitoring of system performance, meticulous contingency planning, persistent trust building, comprehensive audit trails, and unwavering commitment to data quality. Delivering reliable AI is not the end of a journey, but a constant voyage of discovery, innovation, and improvement. Balancing these five pillars of reliability can indeed be a complex task, yet it is an absolutely vital one where AI’s value proposition is considered. By striving for reliability in AI systems, professionals and enthusiasts alike can contribute to more responsible and impactful AI deployments across numerous sectors, harnessing the transformative potential of AI technology.
To explore the other parts in this series, click here.
Recent Blogs
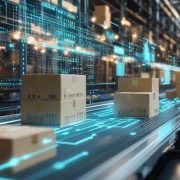
The Future of Supply Chains Is AI-Driven—Is Your Business Ready to Embrace the Change?
July 2, 2025
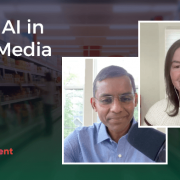
What Retail Media Can Learn from Instacart’s AI Strategy
June 24, 2025
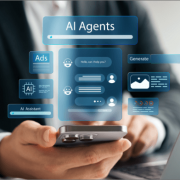
Beyond Chatbots: How Agentic AI Is Automating High-Stakes Business Decisions
June 11, 2025
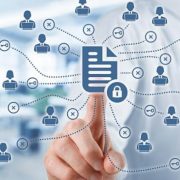
From Batch to Real-Time: The Future of Data Distribution Using Databricks Delta Sharing
June 4, 2025