Explainability is central to the responsible deployment of AI technologies.. It encapsulates the idea that AI systems should not only deliver accurate predictions, but their decision-making processes should be understandable and justifiable for users and stakeholders. Our examination of the topic will include a discussion on how features and data shape AI predictions and explore the significance of human-readable explanations.
Explainability: Building Trust through Understanding
Explainability, at its core, is about making the inner workings of AI systems transparent. It shuns the notion of “black box” AI, which obscures the link between inputs and predictions. This transparency is not merely an academic requirement. It has practical implications in building trust, improving use cases, and complying with regulations that mandate decisions made by AI to be explainable.
“The black box” complexity could potentially lead to unintended and inequitable consequences, particularly in sensitive applications like healthcare, finance, and judiciary systems. With explainability, we introduce accountability, fostering a colocated sense of responsibility and confidence in AI applications.
The Role of Features and Data in AI Predictions
The output of an AI system pivots around the data and features used in its training. Features are variables or attributes chosen as input for the AI model, which based on these, makes predictions. The features chosen and data collected to train the algorithm can significantly impact performance and accuracy.
Consider, for example, an AI system designed to predict patient susceptibility to a particular disease. A well-chosen set of features, such as age, pre-existing conditions, and genetic information, can dramatically influence the prediction accuracy. Similarly, the quality, diversity, and size of the dataset also play an integral part. Faulty, incomplete, or biased data can lead to skewed or unfair predictions.
Human-Readable Explanations: Decoding AI Decision-making
While it is paramount that AI can make accurate predictions, those predictions remain of dubious value if humans can’t interpret them. Human-readable explanations come into play here. Enabling AI to explain its logic in a manner understandable to humans can greatly improve its usability and transparency. Think of it as a translator between the complex mathematical relationships the AI understands and the human language we understand.
Imagine a credit scoring AI that rejects an application. A straightforward “Application denied” message, although accurate, isn’t particularly useful. Instead, a useful response might be: “Your application was denied due to your high debt-to-income ratio and recent default history.” This empowers the applicant with the understanding to improve their credit score.
Explainability is Not an Optional Add-on
The mission of responsible AI frameworks goes beyond accurate predictions. To empower users and build trust in these powerful systems, we must give attention to explainability. Accordingly, carefully choosing features and providing quality data lays the groundwork for predictions made, while fostering an environment where AI can offer human-readable explanation serves as the bridge between machine output and human input.
As we continue to adopt and weave AI even deeper into the fabric of society, it becomes increasingly more critical that we infuse transparency into these systems. Explainability is not an optional add-on, but an essential ingredient for responsible AI, ensuring these powerful tools are accountable, understandable, and ultimately, a force for good.
To explore the other parts in this series, click here.
Recent Blogs
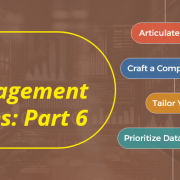
Essential Tips for Telling Data Stories to Executives: Part 6 of Data Management Blog Series
July 24, 2024
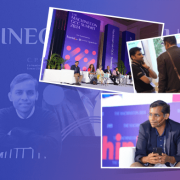
Global Capability Centers Lead the Way in AI Innovation: Highlights from 2024 GCC Summit
July 18, 2024
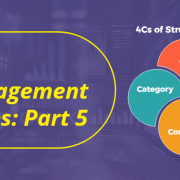
4Cs of Structured Data: Part 5 of Data Management Blog Series
July 11, 2024
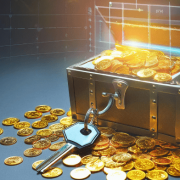
Full Stack Data Products: Bridging the Insight-to-Action Gap
July 9, 2024