highlights a paradigm shift as organizations transition from traditional big data setups to small and wide data models. This transition is crucial for supporting a diverse array of use cases in advanced analytics and AI across various industries. The concept of “wide data” refers to leveraging data in its native format, eliminating the need for complex and time-consuming data pipelines that move data to centralized storage. Data virtualization emerges as a critical enabler in this context, facilitating quick and efficient data usage. Recently, we’ve observed a growing trend among our clients to integrate virtualization platforms into their technology landscapes in order to advance their agility. Gartner research
Why Data Products with Data Virtualization?
While data virtualization accelerates data integration, it presents challenges in data discovery, contextualization, reusability, governance, data quality, security, and lineage. To maximize the benefits of a virtualization platform and meet business needs swiftly and efficiently, it’s advisable to layer data products with data virtualization. This approach helps contextualize data, establish a comprehensive data dictionary within a domain, track data lineage, ensure data quality, provide domain-level security, and enable reusability. Additionally, it defines clear ownership and governance policies.
Understanding the Terminologies
Let’s start by defining the key terms for clarity.
Data Virtualization
An architecture pattern that delivers a unified and integrated view of data for business consumption in an accelerated manner. Key features include:
- Data Federation: Access data from various sources via a single interface.
- Central Security: Implement security and access control at the virtualization layer.
- Faster Time to Market: Accelerate business use case development by reducing data movement.
Data Product
A logical framework designed to address business challenges within a domain context, technology-neutral, offering ready-to-consume data for specific business use cases. Key features include:
- Bounded by Context: The entire data lifecycle is confined within a domain boundary.
- Business Ownership: Business stakeholders own data products, with IT serving as an enabler.
- Discoverable: Data products are cataloged for easy discovery and increased reusability.
The Essential Elements of an Effective Data Product
A data product layered with virtualization strategically leverages data to meet specific business requirements quickly. It is built upon several essential components to ensure the seamless and efficient delivery of data-driven insights and solutions. These components include:
- Virtualization layer grouped into domains to enhance manageability and align data organization with business functions. This approach allows for more efficient data handling within specific contexts, ensuring that data remains relevant and easily accessible to relevant stakeholders.
- Common data model by domain that simplifies the creation of ready-to-consume datasets tailored to specific use cases, enabling business users to derive actionable insights quickly without dealing with the complexities of source data integration and transformation.
- Catalog Integration to improve the reusability of datasets and support self-service capabilities. A catalog ensures that your datasets are well-documented, easily searchable, and accessible to end-users, thereby promoting data democratization and efficient use of data resources across the organization.
- Ready-to-consume insights through embedded analytics within the data products to deliver actionable insights directly to business users, facilitating faster and more informed decision-making processes.
- Cross-domain integration through domain APIs to allow for seamless data flow and interaction between different domains. This capability supports the creation of comprehensive, multi-domain insights and fosters collaboration across various business functions.
- Governance and Security at the domain level to ensure compliance with relevant regulations and internal policies. This approach includes defining clear ownership, establishing governance policies, tracking data lineage, and ensuring data quality and security, protecting sensitive information and maintaining data integrity.
- Monitoring and tracking to optimize platform usage and enhance the customer experience. This will allow organizations to gain insights into usage patterns, identify performance bottlenecks, and make informed decisions to improve the overall efficiency and effectiveness of the data ecosystem.
Ten Tips to Drive Success with Data Products
Creating successful data products requires diligent effort and meticulous design to ensure they deliver the expected return on investment. Here are ten proven strategies to drive success with data products:
1. Leverage Domain Expertise
Collaborate closely with business stakeholders to ensure effective data product creation, utilizing domain-specific knowledge.
2. Select the Right Integration Tools
Choose products that easily integrate existing data sources and consumer applications to minimize implementation time and complexity.
3. Assess Data Granularity
Evaluate the granularity of data across sources to ensure better analytics and alignment with the common data model.
4. Develop a Comprehensive Common Data Model
Plan and execute the development of a thorough common domain data model, recognizing that this process is complex and requires significant domain knowledge.
5. Plan for Transformation Effort
Acknowledge that virtualization tools may not support complex data transformations, necessitating source systems to perform the required data adjustments to fit the common model.
6. Tag Data Entities with Domain Context
Ensure all data entities are appropriately tagged with their respective domains to enhance platform effectiveness and data organization.
7. Implement Reference Data Management
Develop and execute a strategy for managing reference data to support standardization and improve overall data quality.
8. Facilitate External Catalog Integration
If the selected product does not include built-in catalog features, prepare for potential third-party integrations.
9. Monitor Data Product Usage
Implement robust usage monitoring systems to evaluate the adoption of data products and make informed decisions regarding their lifecycle.
10. Consider Scalability and Performance
Factor in scalability and future growth to ensure optimal cost and performance of the chosen virtualization platform.
Elevating Business Outcomes with Infocepts
Infocepts brings a wealth of experience in implementing data products, covering everything from landscape assessment and tool selection to comprehensive solution implementation and support. Our innovative solutions such as Decision360, streamline the setup of data product ecosystems, delivering actionable insights from your data.
Learn how we can help you transform your data landscape and drive your business forward.
Recent Blogs
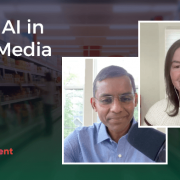
What Retail Media Can Learn from Instacart’s AI Strategy
June 24, 2025
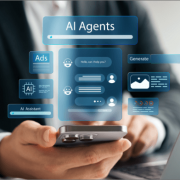
Beyond Chatbots: How Agentic AI Is Automating High-Stakes Business Decisions
June 11, 2025
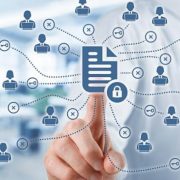
From Batch to Real-Time: The Future of Data Distribution Using Databricks Delta Sharing
June 4, 2025
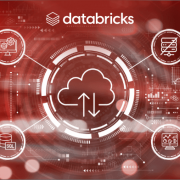
Accelerating Databricks Serverless Adoption with Agentic AI & Proven Practices
May 29, 2025